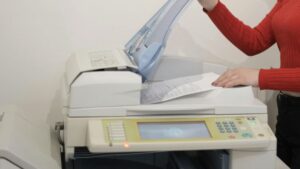
With the New York Times vs. OpenAI trial moving along, we might see the first cases on AI training material and copyrights. The courts will decide whether AI learning is a fair use of copyrighted material. The verdict will impact everyone who creates content. Whether you make videos, blogs, or social media posts, the court’s decision will significantly affect how we conduct ourselves as persons and as businesses. However, the verdict might go beyond AI training. During the Q&A section of my recent LibrePlanet talk, the discussion moved into whether AI training is close to human learning. An affirmative answer would consequently implicate our ability to learn and process knowledge. After all, when we read the newspaper, we filter out the relevant bits, both in information and the language arts, and enhance our understanding. When needed, we recombine the bits and pieces with older information. It sounds similar to AI; let’s find out.
Recombining Knowledge in Learning
In its most simplistic form, learning is the process of taking external or internal stimuli and transforming them into new neural pathways within the brain. Listening, touching, or reading are examples of external stimuli. Thinking, remembering, problem-solving, and decision-making all give us internal growth catalysts.
On the surface, AI training is very similar. Data provides external stimuli, similar to reading. The stimuli are then weighed and turned into new parts of the model. Additionally, the retrieval process and external feedback on the results can serve as further triggers to enhance the model.
One significant difference, however, is the snippets we retain and how we combine them with others. For example, the poem Invictus by William Ernest Henley contains the line “How charged with punishments the scroll.” This line always reminds me of the Thieves Guild storyline in the Computer Game The Elder Scrolls Oblivion, two things that admittedly have very little in common.
Our brain is filled with these strange cross-connections that don’t make sense on the surface. Yet, they help us adapt to new situations and social queues.
Creativity is Making a Difference
The difference between our brain and the Deep Learning Models in AI is most apparent in creative activities. Even if we had never heard or seen a phrase or word combination before, we could come up with it. AI, on the other hand, is built using mathematical models. Something that didn’t exist beforehand has a zero probability of being created unless a programmer manipulates the model.
Thus, wrong answers either come from deliberate manipulations, like Google’s diverse Nazi soldiers, or from faulty training data, like recommending glue to secure pizza cheese. They don’t come from an inherent creative drive to try out new things and analyze the results.
Yet, this creative part of our thinking and learning sets us apart from AI training. We can develop through processes beyond pure logic.
Background Questions in the Case
Apart from the general differentiators between AI training and learning, other policy questions are involved in the debate. No matter how the courts decide the current cases, we are likely to continue debating them while our understanding and usage of AI grows.
The underlying problem is that we never had a mechanical or electronic system that could create derivative works or completely new works based on millions of data sources. Thus, some problems are fundamental to how we want AI to develop.
- LLMs are trained on thousands of works with similar inputs, making it difficult to determine the source. Thus, when is something a source, and when is a copy?
- When does the violation occur? Is it when creating the AI model, using it, or publishing something AI-related?
- How far does “Fair Use” go in the case of AI?
- Who is responsible for checking for copyright violations? Is it the user or the developer? After all, the Betamax case absolves the manufacturer of many responsibilities.
Learning: a Case for Legislation
While the big picture of learning and AI training appears very similar from 50.000 feet, the details are very different. Yet, the question remains: will the courts be able to differentiate between the two in finding a just verdict, or will they go too far one way or the other?
This dilemma shows that we shouldn’t leave the differences between learning and AI training to the courts. Our legislators should decide the fundamental policy questions. Thus, it might be time to revisit the copyright law and determine how it needs to evolve to handle the current wave of AI-based products. Otherwise, we might find that a court suddenly disallows human education because, on a very high level, it is too similar to an AI copyright violation.