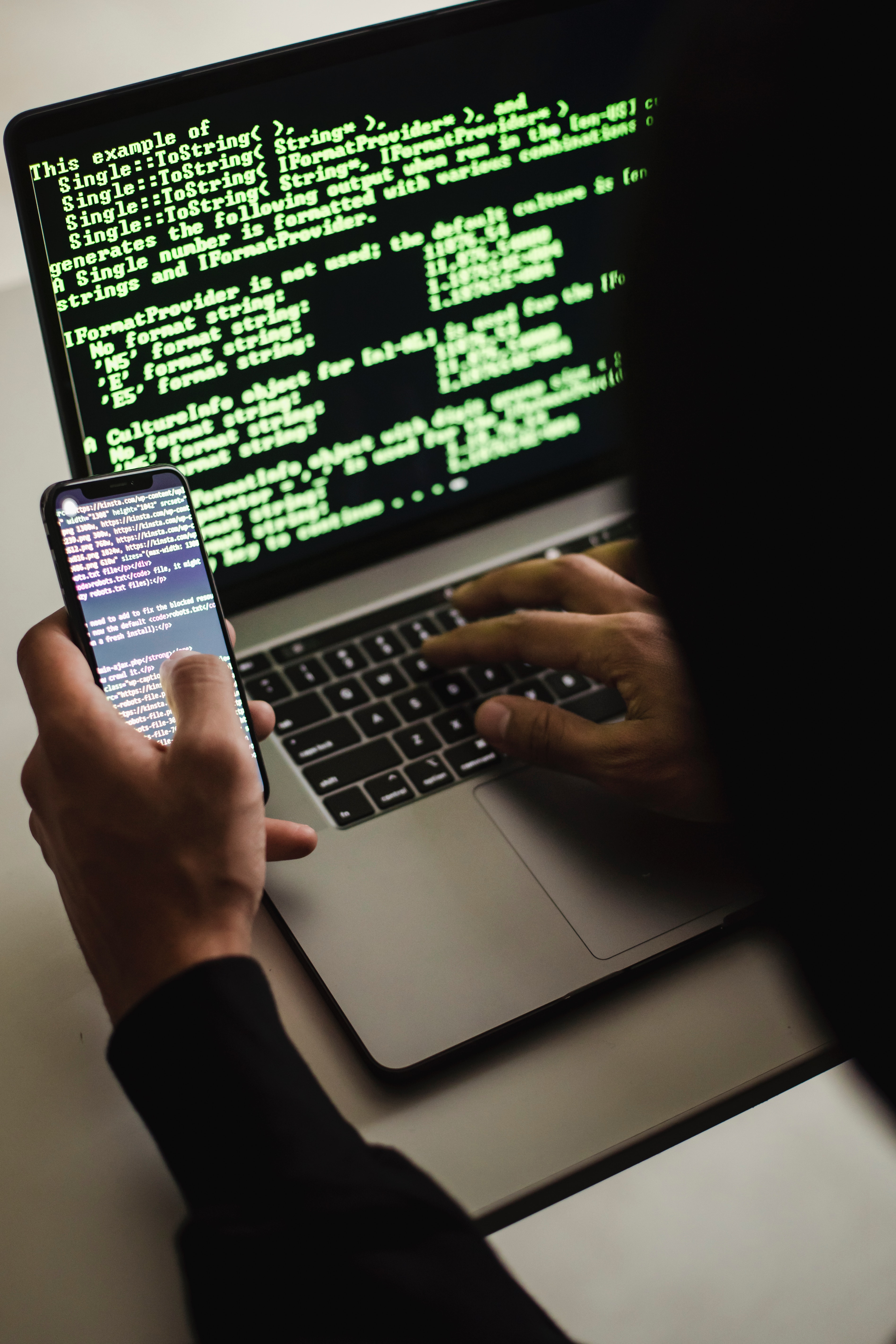
With the release of ChatGPT, Artificial Intelligence and large language models (LLMs) have arrived at our fingertips. Microsoft’s integration of ChatGPT into Windows, and the possibility of Apple following suit, will bring it closer to millions of users who don’t use free software. Yet, AI has some severe shortfalls that remain unaddressed. Reasons not to use AI are plentiful, from legal risks and security concerns to biases and questionable accuracy.
Let us dive into why you should ban AI from your shadow IT and regulate any sanctioned uses.
Legal Risks and Responsibilities
The past twelve months have shown numerous legal risks when training and utilizing LLMs. Book authors and the New York Times have filed copyright lawsuits against OpenAI. GitHub’s Co-pilot, meanwhile, is liberally copying Open-Source code and might open up companies utilizing it to copyright violations.
Yet, it isn’t only copyright law that could impact AI utilization. AI regulations across the world pose another significant threat. As with any fast-moving technology, the regulation can barely keep pace. Companies must stay on top of it if they plan to build products based on Artificial Intelligence.
Lastly, data privacy and data protection will play a significant role. Errors could lead to a considerable headache, especially for companies or employees using customer data and interactions to refine their models.
AI Security Concerns
Beyond the legal ramifications, the cybersecurity issues around utilizing customer data are a problem in their own right. Especially if AI becomes part of the shadow IT, a technology used by employees without authorization, there will be instances where LLMs exposee private or proprietary data to the internet.
Spoofing of popular AI websites is another attack that IT management should not ignore. Exposed to performance pressure, employees might copy data from a fake website.
Lastly, the vast data stores of any model make it an excellent target for ransomware attacks and data exfiltration. Companies spend hundreds of dollars to train models. Thus, they might be more willing to pay a ransom or to stop criminals from exposing the model.
Productivity Impact Using AI
The ability to ask LLMs to answer any question is a double-edged sword. It can quickly provide an answer and a summarization to any question. At the same time, this ability can quickly lead you down the rabbit hole where more research on a person’s background and life events will help you finalize the report.
It is similar to the Wikipedia game, where you try to get from one random article to a different one by clicking links in an article.
When officially introducing AI, you can counteract it with the appropriate training and instructions. Shadow IT unfortunately lacks these instructions.
AI Accuracy
Today, we train LLMs and GenAI with various sources from the internet. Everything goes into the model, from books to news articles, without filtering, review, or accuracy checks. Every answer relies on this collection of works.
Yet, the models are neither aware of the context of the source material nor the context of today’s question. Consequently, answers may be widely outdated or inappropriate for the requester’s situation.
While the answers may be obviously out of date in some cases, in others, it would require careful verification. Yet, with AI talking almost humanlike, our brain wants to trust this perceived expert.
Yet, this only touches the wrong answers due to incorrect or outdated source material. There are enough instances where AI made up material when it didn’t know a fitting answer.
While there are ways to get the model’s citations and references, users must be aware of the issue. Again, shadow IT without training lacks this awareness.
AI Biases
AI tools learn from the past without understanding the context of the past and the developments since. Consequently, they are likely to internalize biases and patterns that are outdated and discriminatory. While some are obvious, like one AI suggesting the only suitable employees are called John and play water polo in college, many times, the patterns aren’t as obvious.
In many fields, but particularly HR, anti-discrimination laws are strong. Ensuring no implicit biases or active discrimination differentiates a thriving company from a multi-million dollar lawsuit.
Yet, AI biases play a role beyond hiring. Suppose they score sales opportunities based on faulty criteria. In that case, e.g., the gender of the requester, the sales department might pursue the wrong opportunities.
Consequently, AI needs careful checks for biases. We must review it for past societal discrimination and implicit preferences in the data.
Building Checks and Policies
AI can have an enormous potential to transform the workplace. Yet, AI presents a significant hazard without careful planning, thought-out policies, and employee training. Let us ensure that our companies are ready to counter the threat by building workable AI policies and banning the shadow use of the software.